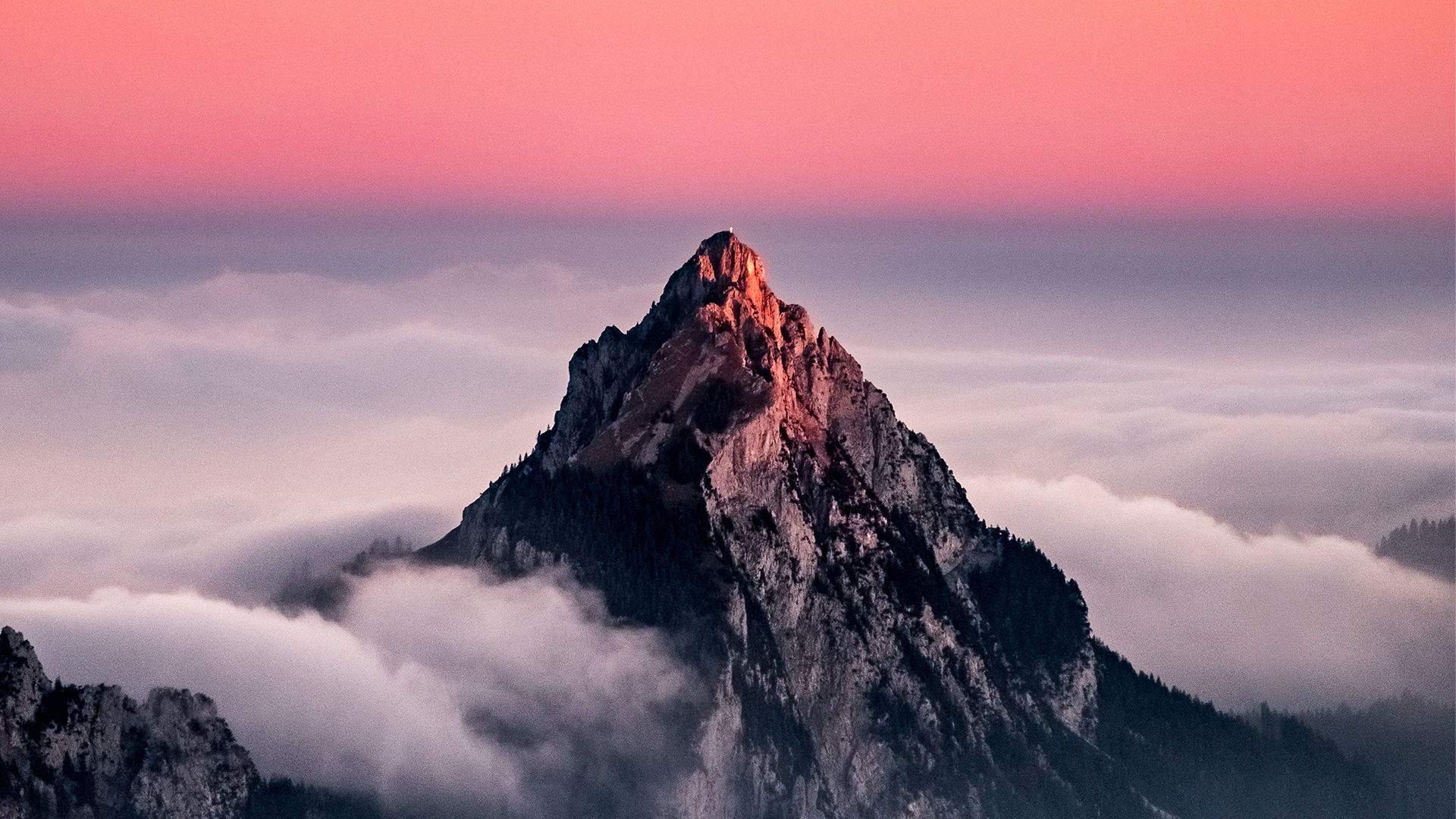
Search Results
228 items found for ""
- Tech Meetups: Working Remotely, Connecting Locally
Analytics is a rapidly evolving discipline. Staying up to date with the latest methods and software can be a daunting task to undertake alone. Local meetups provide access to a community of professionals with similar goals. In particular, Meetup.com is one site that supports self-organizing groups, which generate events based on a common interest. In the tech space, these can range from networking events to book clubs and hackathons. In the D.C. area alone, there are over 100 meetup groups related to analytics and data science. In this post, three CANA team members, Lucia, Walt, and Jerome, discuss how they use meetups to learn, network, and give back to their communities. Lucia Darrow | I use meetups primarily to learn. One of my favorite data science meetups in the Vancouver area hosts monthly briefs based on the online competition website Kaggle. Local teams present a Kaggle competition, share their approach, and then explain the methods of the highest-ranking teams. These presentations give me a chance to think about how to handle diverse problems, often with different data types than I work with in my day-to-day tasks. The combination of algorithms crafted by the winning teams are fascinating! One of my top takeaways is the relative success a simple method can have against these highly complex solutions. Another way I use meetups is to connect with other women and gender minorities in tech, through groups like RLadies and PyLadies, that aim to give minorities a voice in tech communities. RLadies is an organization promoting gender diversity in the R community with meetup groups in 131 cities worldwide. You can check out a map of current locations here. For me, this group provides a bridge to the larger R community and connection to wide range of experienced R users. Hadley Wickham speaking at an RLadies Meetup, August 2018 Walt DeGrange | One of the great things about living in the Research Triangle is the abundance of tech companies. SAS, RedHat, Lenovo, Cisco, IBM, and many more call the Triangle home. It also has three major universities with the University of North Carolina, Duke, and NC State and lots of smaller schools. Given this abundance of analytics, the area boasts many Meetup.com groups. There are groups for languages like Python, R, SAS, and Julia and techniques such as machine learning and AI. There are even groups specific to certain genders like the R-Ladies RTP. In May 2018, the Research Triangle Analytics group ask me to speak. The venue was the SAS Training Center in Cary, NC. The facility was state-of-the-art and had more screens than I had ever seen in one room. I shared three stories from my past that focused on challenges in implementing analytics solutions. There was an excellent discussion after my presentation and several analysts shared their personal experiences. "I love interacting with this diverse group of analytical professionals. The meetups give someone that works at home an opportunity to interact and share in person with other analysts. It also allows me to see what cutting edge in other industries. The cost of getting started is a little time to log into Meetup.com and search for analytics. As an additional bonus, many of the meetings are sponsored by organizations that supply pizza for the evening gatherings." - Walt DeGrange Jerome Dixon I I use meetups to learn, network, and collaborate where it fits with like projects or technologies I’m interested in. My focus is machine learning, becoming a better programmer, and keeping up with the myriad of tools and techniques constantly getting introduced into the data science and technology space. One of the benefits to living in Richmond are the tech companies that sponsor meetups and examples of the use cases that they have actually put into production. I’ve seen amazing presentations by CapitalOne for how they use machine learning and hints into their data engineering infrastructure. I’ve sat through a very novel idea and use case for Tableau Server as basically an extract, transform, and load (ETL) tool by CarMax. Ippon has hosted some great meetups with how they are using Apache Spark as well as some best practices for their IT project management. Below picture is from NVIDIA’s Data Scientist, May Casterline, on the work they are doing with image processing, deep learning networks, and GPU dataframes. Leading edge technology! And when I get stuck - PyRVA (python user group), RVA R User’s Group (R statistical programming language), RVA Linux User Group (Linux, Amazon Web Services), and Docker Richmond meetup group (for containerization). Great opportunities for both support and networking with Richmond’s local meetup community. I am very fortunate and grateful for the city I live. If you are lucky enough to be located in a great meetup community - please leverage! *CANA Advisors is a veteran and woman owned leading logistics and analytics agency based out of Gainesville VA USA. For more information about CANA Advisors and its world class team visit canaadvisors.com #LuciaDarrow #WaltDeGrange #JeromeDixon #techmeetup #meetupcom #kaggle #RLadies #PyLadies #python #R #NVIDIA
- Why the BOM Gives Us a Headache
One of our DoD clients inquired on where they could apply machine learning to improve their repair processes. What makes this process challenging is that repair personnel maintain a list of parts required to do a specific repair task. If for example, your car needs an oil change, then the list of parts for the maintenance would include one oil filter and a few quarts of oil. If the list of parts were always the same, then determining the list would be trivial. Unfortunately, there is always the possibility of performing scheduled maintenance and finding other parts that require repair. Also, there is the additional complexity of having parts replaced by newer parts. When you scale this up to a fleet of thousands of vehicles and tens of thousands of repairs per year, determining the required part list from maintenance personnel experience alone is a daunting task. We recommended an innovative approach using natural language processing (NLP) for creating a Bill of Material (BOM). What is a Bill of Material (BOM)? A Bill of Material (BOM) is a breakdown of items with associated configuration data needed to repair a subassembly or component of a larger system. Usually, these are broken up into a manufacturing domain and a supply chain domain, but there may be additional domains depending on the complexity of the part and the processes needed to repair or procure. Figure 1: The BOM connects the critical domains for a system's supportability Oleg Shilovitsky has written several blog posts and presentations on the challenges of BOM management. His presentation provides a backdrop on the issues that make BOM management challenging. http://beyondplm.com/2016/03/08/pi-munich-presentation-develop-single-bom-strategy-3/ What drives our analysis? Operational requirements drive how many vehicles are ‘Awaiting Repair’. Accurate onhand inventory (BOM Forecast) and ‘Repair Rate’ drive the number of ‘Repaired Vehicles’ delivered. Figure 2 is a high-level depiction of our repair process. Figure 2: High-Level Repair Process We first match the number of ‘Repaired Vehicles’ to the ‘Repair Type’ performed over a set period of time. Our set period of time is typically the previous repair schedule. After we match the repair types completed to the number of issues per vehicle, we then calculate the frequencies of the parts ordered. Our final step is to divide the frequency counts by the number of vehicles per period to get a Replacement Rate (RR) per individual part. This RR with a part number now becomes our forecasted Bill of Material (BOM). Natural Language Processing (NLP) Refresher A term document matrix is a way of representing the words in the text as a table (or matrix) of numbers. The analysis uses the rows of the matrix to represent the text responses, and the columns of the matrix to represent the words from the text. Once in a term document matrix format, we can apply various text-mining algorithms. Figure 3 provides an example of this process. Figure 3: NLP Bag of Words Part numbers or National Item Identification Numbers (NIINs) can be represented as words or character vectors for text mining analysis. By representing this as a text mining problem, we gain efficiencies in computer memory utilization in addition to additional analysis methods. Job Order Number (JON) refers to the type of repair action and the required parts and procedures required for repairs. Control Order Number (CON) refers to the higher level JON that a major repair action falls under. CONs and JONs will be our “bags” for collecting words (NIINs). Figure 4 shows a high-level view of how JONs (sentences) are made up of NIINs (words). Figure 4: Parts Data in Array Format BOM Building Example We use R to create the required data structure and perform the frequency analysis. We format our issue data into a list array by Repair Type: Figure 5: Issue Data in List Array Data Structure Figure 6: Issue Data – expanded We then convert our list array to a document term matrix, count frequencies, and calculate the individual Replacement Rates. Figure 7: Final BOM BOM Building Made Easy Here we have shown a relatively easy, data driven approach to developing a BOM. Our goal is to reduce the workload of the repair personnel to perform BOM maintenance and create a more proactive approach to BOM management. By also monitoring repair rate trends from period to period, maintainer or senior management can identify missed configuration changes or possible changes to local repair procedures. These techniques can be applied to the manufacturing side as well. This article was written and developed by the creative team of analysts at CANA Advisors. Jerome Dixon is a Senior Operations Research Analyst at CANA Advisors jdixon@canallc.com Aaron Luprek is Senior Software Developer at CANA Advisors aluprek@canallc.com Walt DeGrange is the Director of Analytics Capabilities at CANA Advisors wdegrange@canallc.com #NLP #DoD #BOM #billofmaterial #naturallanguageprocessing #JON #jobordernumber #JeromeDixon #WaltDeGrange #AaronLuprek
- 2018 86th MORS Symposium
CANA at the 86th MORS Symposium Professional societies are a way to network, share knowledge and techniques, and move the profession forward. CANA Advisors contributed in a big way to this goal during the 86th MORS Symposium in Monterey, CA at the Naval Postgraduate School. CANA's Norm Reitter, Lucia Darrow, Carol DeZwarte, and Walt DeGrange CANA's Carol DeZwarte continued to lead interesting and well attended sessions in Working Group 17: Logistics, Reliability and Maintainability as a co-chair. She will be fleeting up as the chair of the working group next year. This group is considered the home working group for many CANA analysts who have attended and briefed much of their work there over the past years. There were many great briefs covering everything from optimizing inventory to how to develop and deploy complex analytical models. Two sessions covering how CANA used R to capture inputs and present outputs for a large discrete event simulation attracted huge audiences. CANA's Lucia Darrow did an excellent job discussing the tech behind the implementation and emphasized the importance of deliberate design. She presented "Force Closure Model (FCM): Decision Support Tool Orchestration in R" in Working Group 10: Joint Campaign Analysis and "On-Demand Custom Analytics in R" in Distributed Working Group: Emerging Operations Research. Lucia Darrow of CANA Advisors presenting at the 86th MORSS CANA's Walt DeGrange participated as a panel member in a standing room only ethics in analytics discussion. The session discussed the special responsibility that each analyst has to represent their unbiased mathematical model to the best of their ability. There were also several ethical dilemmas that were posed by the audience for the panel to discuss. CANA's Norm Reitter chaired a meeting of the new MORS Logistics Community of Practice. Close to 30 MORS participants attended to discuss the latest issues and possible solutions within the National Security community. This symposium also saw the departure of Norm and Walt from the Board of Directors. Norm finished up six years on the board serving as MORS President and finishing up as Past President this year. Walt finished out his four year term on the Board of Directors as the Vice President of Professional Development. Both will remain very active in teaching for the MORS Certificate Program (MCP), and Norm will help future board members in his role as an Advisory Director. Overall, a great week of networking, learning, and collaborating with the Military Operations Research community. #86MORSS #CANAAdvisors #NPS #MORS #2018 #MORS #symposium #CarolDeZwarte #WaltDeGrange #NormReitter #LuciaDarrow #86MORSS #NPS #R
- A CANA Congratulations!
It is our pleasure to announce the promotion of Norm Reitter to Chief Analytics Officer/Senior Vice President of Analytics Operations at CANA Advisors! Norm has served successfully as CANA’s Director of Analytics since January 2014. Since 2014, he has dedicated himself to building and managing a diverse, dynamic team of operations research analysts, software developers, statisticians, graphic artists, and subject matter experts who together provide innovative and “usuable” solutions to CANA’s commercial and governmental clients. Norm has distinguished himself as a key advisor to CANA during this time – providing insights and input into the company’s strategic growth and market expansion. As he takes on this new executive dual role, Norm will develop and manage CANA’s Information Technology (IT) and Independent Research and Development (IRAD) programs, advise on future analytic investments and offerings, and lead CANA’s rapidly growing Analytics Operations services line. Norm boasts over 25 years of military and commercial experience providing logistics & analytics expertise and solutions. He holds an undergraduate degree from the U.S. Naval Academy and a graduate degree in Operations Research from the Naval Post Graduate School in Monterey, California. He currently serves in leadership roles in several professional analytics organizations. He is the Immediate Past President of the Military Operations Research Society (MORS) and the chair of the Analytics Capability Evaluation (ACE) Sub Committee with the Institute for Operations Research and the Management Sciences (INFORMS). He has three highly accomplished children – Summer (currently working towards a PhD in Psychology at Indiana University of Pennsylvania), Madison (a senior graduating this June and attending Chatham University in the fall pursing a degree in Sustainability), and Josh (entering his senior year in high school this fall). When he is not leading all things Analytics at CANA Advisors and raising three amazing young citizens, Norm is snow shoeing, hiking and paddling in the mountains and lakes of Colorado. Please join us in congratulating and welcoming Norm to this new position! #congratulations #promotion #CANAAdvisors #NormReitter
- CANA Members “2017 Give Back Day”
CANA Advisors through its CANA Foundation – supports our people and offers opportunities to ‘give back’ in many ways. One specific form of support this past 2017 holiday season was to give our team members company time to spend “volunteering in their local community.” To quote our company’s Founder and President, Rob Cranston, the CANA Foundation “provides the CANA family of employees an opportunity to connect with and give back to community areas we feel passionate and care about.” Below are a few stories of how our team members chose to ‘give back’ using this time. Bicycles for Monterey Principal Operations Research Analyst Harrison Schramm used his volunteer time in support of a project with Monterey County Behavioral and Mental Health – procuring and providing bicycles for kids in need. This project started several years ago in a casual conversation between Harrison and the Project’s leader. She knew that Harrison was in to riding bicycles and wondered if he could help build a few. One thing led to another, and he ended up with a wrench in his hand the week before Christmas 2016. Clinicians in contact with families provide a list with information such as age, height, and gender. An anonymous donor contributes money. Harrison and a few others convert the money into age appropriate bicycles. The clinicians then pick up the bicycles and deliver them to the families. The process is ‘double blind’ in the sense that the providers and recipients of the bicycles will never be introduced. Harrison completing the 2017 Bicycle Build: Six bikes and one Scooter. “That doesn’t stop me from wondering, though” Harrison said. “Sometimes, I’ll be out on the Rec-trail, see a kid coming and wonder ‘did I build that bike?’” The bicycles are all brand-new, and a helmet is provided with each. “A bicycle isn’t just a toy for a kid on the [Monterey] Peninsula. It’s exercise, it’s a way to get to school and work, it’s a way to put everything behind you – if only for a few minutes.” Harrison says that he prefers to get the bicycles un-built from stores if he can, because he can fit more in his car that way. Kitsilano Neighborhood House Operations Research Analyst Lucia Darrow spent her volunteer hours at the Kitsilano (“Kits”) Neighborhood House, helping out with the Kits Club after-school childcare program. The Kits House develops programs to meet the needs of the community, ranging from childcare and senior living options to hosting farmers markets and ESL circles for newcomers to the city. Through volunteering with the Kits House and assisting with special events, Lucia says she enjoys connecting with the community and learning about the rich history of Vancouver’s Westside. Lucia on the steps of the Kitsilano Neighborhood House. Samaritan’s Purse Operation Christmas Child Norm Reitter, our Director of Analytics, spent an afternoon at a Samaritan's Purse run "Operation Christmas Child" gift distribution center where he inspected and enhanced gift boxes that were collected from many donation sources. These boxes were then routed through the Denver, Colorado distribution center and shipped to children in need who would not otherwise get Christmas gifts. Operation Christmas Child counts on thousands of volunteers to collect and process millions of shoebox gifts every year. Samaritan's Purse provides this approach so that kids get meaningful and useful Christmas gifts. Norm and Annalisa were busy inspecting donations, adding age appropriate items to gift boxes, and packing the gift boxes into larger containers for shipping. Norm said that seeing all the donations and knowing the positive impact on each child that would receive a gift box made this a very meaningful experience for him and Annalisa. Norm and Annalisa at their local Colorado Operation Christmas Child gift distribution centers. In Closing CANA Foundation has enjoyed a wonderful inaugural year of growth and giving back to our communities. We are excited to continue our upward momentum and build upon that success. In 2018, we will continue to create more opportunities for our team to participate, facilitate our team’s ideas to give back, and continue to develop meaningful relationships with other organizations. Onward and upward!! If interested in learning more about the CANA Foundation or in partnering with us, please reach out to Kenny McRostie, our CANA Foundation manager, at kmcrostie@canallc.com. #CANAFoundation #CANAAdvisors #givingback #charity #community #support #bicycles #Kitsilano #KitsHouse #Samaratin #operationChristmasChild
- Using the SEAL Stack
Recently, we needed to develop a desktop application for one of our clients. As web developers, our immediate thought was to use the SEAL Stack (http://sealstack.org). SEAL is a technology stack that uses SQLite, Electron, Angular, and Loopback. Why use Electron? Electron (https://electronjs.org) gives a developer the ability to build cross platform desktop apps with JavaScript, HTML, and CSS. It is a framework developed by GitHub. It combines Node.js, which is a JavaScript runtime that allows you to run JavaScript on the desktop, with Chromium, the open-source technology behind Google’s Chrome browser. This allows a developer to develop as if it is a web app, but from the user's perspective. It functions as a single application. Electron is used in many popular applications including Slack, Microsoft Visual Studio Code, and tools from GitHub. Why use Angular? Angular is a front-end web framework developed by Google. (https://angular.io). It makes writing single-page apps easy. It uses declarative templates for data binding and handles routing. It promotes component reuse across your application, making your code more stable. Angular uses TypeScript, an extension of JavaScript that adds strong typing. Running a Web Server An interesting twist to the project, was that there was a high probability that the client would want it converted to a web app in the future. Aside from the benefit of being able to develop with familiar web technologies, Electron gave us the ability to easily transition to the web at a later date if needed. With this knowledge in mind, we decided from the very beginning to build the app like a standard single-page app and use Electron to run it. Because Electron runs on Node.js, it was easy to spin up a server within the app. In the future, if we need to transition the app to the web, it will simply require deploying the code to a web server (and a few additional tasks such as changing data connectors to connect to a database server, adding authentication, etc). Why use LoopBack? For the web framework, we chose LoopBack (https://loopback.io). LoopBack is a highly-extensible, open-source Node.js framework. It is built on Express, the most popular Node.js framework. It makes it easy to quickly create dynamic end-to-end REST APIs. It has an ORM and data connectors for all the standard databases making it very easy to retrieve and persist data. Why use SQLite? By default, the LoopBack boilerplate configuration uses memory for data storage. Because we needed the data to persist between sessions, so we decided to use a database for data storage. In this case, we chose SQLite (https://sqlite.org). Benefits of SQLite include not having to install a database server on the user’s computer. SQLite is public domain and works across many different platforms. The data is stored in a single .sqlite file that can be transferred from one computer to another if needed, which could help with syncing data between users in the future. To avoid any issues running SQLite cross platform, we used a Node.js implementation of a SQL parser called sqljs. and wrote a custom connector for Loopback using sqljs (https://github.com/canallc/loopback-connector-sqljs). System Architecture Here’s a diagram illustrating how the four elements of the SEAL stack integrate together. Wiring it Up The easiest way to get started with the SEAL stack is to use the quick-start project (http://sealstack.org). The site is well documented. It also provides instructions for modifying an existing application to use the SEAL stack. This article was a collaboration between CANA Advisors Principal Software Developer Dan Sterrett, and CANA Advisors Senior Software Developer Aaron Luprek. For more programming articles, information on on SEALstack and other projects in development visit CANAadvisors.com #SEALStack #stack #SQLite #Electron #Angular #Loopback #framework #developer #desktopapp #JavaScript #TypeScript #WebServer #AaronLuprek
- How Learning French Refreshed My Analytical Strategy
A few months after graduating with an advanced engineering degree, I find myself back in the classroom, this time for my first class of beginner French. All about me I hear snippets of broken French from my Canadian classmates: phrases, simple sentences and questions. I know three words, which I can pronounce in a distinctly American way: bonjour, merci, and croissant. The “beginner” level of French language for Canadians, it turns out, is a little different from the “beginner” level for an American. I reassure myself that I’m a fast learner and struggle through the first class. After years of focus in one area of work, it’s natural to grow confident in your carefully crafted method of learning and doing. Once varied problems start to take on familiar forms, and it becomes easier to prescribe a certain solution. Stepping into French, I realized my tried and true approaches to learning were not going to prove effective. Several months later, here are some lessons I learned. Failing: Fast and often. I find the most difficult part of language acquisition is not grammar or syntax, but the inevitability of mistakes. Regarding mistakes as taboo creates a major roadblock to personal improvement. The same holds true with solving a difficult analytics problem. Instead, sharing in-progress or flawed work with colleagues helps to break through the small failures and clear a path to a robust solution. Out with the old and in with the new – Suppressing instinct and embracing a new technique. As with many language learners, my first instinct when I don’t know a word is to simply throw in the word from another language. Similarly, we tend to retain old sentence structures, until the structures of the new language become natural. R users can understand how this relates to learning the dplyr workflow or transitioning to functional programming. While these changes feel like a major paradigm shift at first, the impact on future work can prove invaluable. Analytics MacGyver. Asking someone about their aunt’s profession can sound something more like “What does your mother’s sister do in life?” coming from a novice speaker. This roundabout method may sound silly, but is arguably better for the learning process than simply inserting words in English. Analytics professionals must also be bricoleurs, utilizing many resources, tools, and experts to make complex and unfamiliar problems tractable. Diving in and staying in. Immersion and persistence are key to language acquisition. In analytics, methods are rapidly changing and improving. Attempting to become proficient in every new technology can be tempting, but dedicating time to one technology allows for quicker mastery. Abstraction and derivation of meaning. In the early stages of learning, every interaction with a new language can feel like a game of abstraction, as we try to translate back to our mother tongue. As sentences become phrases, then complex sentence structures, the problem becomes a greater puzzle. Here is where I’d argue that many analytics professionals would find joy in the challenge of language acquisition - the feeling of successfully working through a verbal puzzle and constructing a response, hopefully more expressive than oui or non. Lucia is an Operations Research Analyst at CANA Advisors. To find more content on learning and leveraging analytics, continue to visit our CANA Connection. #learningFrench #strategy #Analytics #LuciaDarrow #R
- Fake News: A Problem for Data Science?
Over the past year, "fake news" has become a topic of particular interest for politicians, news media, social media companies, and... data scientists. As this type of news clutter becomes more prevalent, individuals and organizations are working to leverage computing power to help social media users discern the "fake" from the legitimate. In this article, we take a look at some basic natural language processing (NLP) ideas to better understand how algorithms can help make this distinction. Natural Language Processing: A Brief Introduction Text Preprocessing: Arguably the most important step to text mining is preparing the data for analysis. In NLP, this involves actions such as tokenizing words, removing distinctions between upper and lower case words, stemming (extracting the root of words), and removing stop words (common words in a language that don't carry meaning-- think: the, and, is). An example of tokenization and stemming is shown below in Figure 1. Bag of Words: This model is useful in finding topics in text by focusing on word frequency. Bag of words can be supplemented with word vectors, which add meaning to NLP representations by capturing the relationship between words. Text as a Graph: Graph-based approaches consider words as nodes and focus on associations to draw more complex and contextually rich meaning from text data. Named Entity Recognition (NER): This method can be used to extract types of words, such as names, organizations, etc. Many NER libraries are online for public use. Sentiment Analysis: Otherwise known as "opinion mining," this technique provides a gauge of the author's feeling towards a subject, and strength. Do fake news outlets produce more opinionated articles? # Tokenization and Stemming Example headline <- "The Onion Reports: Harry Potter Books Spark Rise in Satanism Among Children" tokenize_word_stems(headline) ## [[1]] ## [1] "the" "onion" "report" "harri" "potter" "book" ## [7] "spark" "rise" "in" "satan" "among" "children" Figure 1. Tokenization and Stemming Example How Are Data Scientists Framing the Problem? While popular browser extensions use crowdsourcing to classify sites that publish fabrications, researchers are reframing the problem of fake news. In order to fit a model, an understanding of the most influential features that differ between fake and legitimate is helpful. Regardless of whether the fake news is created by provocateurs, bots, or satire, we know it will have a few things in common: a questionable source, content out of line with legitimate news, and an inflammatory nature. Current research in the area takes advantage of these truths and applies approaches spanning from naive Bayes classifiers to random forest models. Researchers at Stanford are investigating the importance of stance, a potential red-flag trait of misleading articles. Stance detection assesses the degree of agreement between two texts, in this case: the headline and the article. Another popular approach is the use of fact-checking pipelines to compare an article's content to known truths or an online search of a subject. As the complexity of fake news adapts to modern modes of media consumption, research in this space will expand. Image classification is a likely next step, albeit one that poses a major scalability challenge. Interested in learning more or building your own fake news classifier? Check out these resources: Python's Natural Language Processing Toolkit R's NLP Package Python's SpaCy for NER Our analysts at CANA Advisors are always interested in hearing from you. If you have an interesting “data” dilemma, contact Lucia Darrow. [EMAIL] #fakenews #science #NER #NLP #NaturalLanguageProcessing #tokenization #stemming #datascience #LuciaDarrow
- What I wish I had known then - An excerpt from article appearing in OR/MS Today
Background: This article came about from a series of discussions between CANA’s Harrison Schramm* and MORS** Director and NPS Faculty Member Captain (USN) Brian Morgan, culminating in an one-off lecture on 24 August 2017. After receiving several requests for slides, Harrison and Brian decided that it would make more sense to simply write an article, which appears in the October, 2017 issue of OR/MS Today ***. Below is a short summary of the original piece. In our Profession we stand on the shoulders of Giants, but one cannot expect to get there without a ladder. In summary, we identify the following bolded as the key insights: Do Work That Matters Consider the following ‘quad chart’ of importance and difficulty: Figure 1: Your professional life. If you find yourself blessed to be in the top left quadrant, congratulations, stay there as long as you can. If you find yourself in the lower right corner, get out of there fast! If you find yourself doing work that is both important and challenging, congratulations! Savor that moment, because it is our experience that if you can spend 15 percent of your time in that quadrant you should count yourself blessed. Work That Doesn’t Matter: Feeding Pigeons No matter how good you are, or how hard you try, you will find yourself occasionally in the “not challenging, not important” quadrant. We offer two possibilities: First, work that is not interesting can be made interesting by being a test bed for a new programming language or a technique. This is like Mr. Miyagi in “The Karate Kid” using “wax on, wax off,” turning the mundane task of polishing the car to training for competitive karate. The second possibility is more nuanced: look for a problem that is important using a similar technique and apply what you’ve learned. There are at least three “keys” to doing work that matters: 1. An important question. It turns out that no matter how elegant a statistical model of washing our socks we build, it will never be top-tier work. This is because it is a question that nobody cares about! The first, key ingredient to having important work is to work on an important question. 2. Quality data. No data set is perfect. Quality data – that stakeholders respect – is necessary and time should be devoted to it. 3. A proponent. Perhaps the most important factor, and the most elusive. A proponent is a human being, usually not an analyst, who has the authority to take the work you have done, turn to the people who run the system under test and say, “Go do what these folks just recommended.” Collaborations and Teamwork We cannot think of any worthwhile pursuit that is done totally alone. Even if one were a walking O.R. encyclopedia, they would still need peer review to avoid the intellectual “echo chamber.” Unsurprisingly, good teamwork, clear and concise communications, and meeting goals are so valued in colleagues. A good teammate is a good teammate. Focusing on What’s Important This means taking some time each day and dedicating it to the state of the practice. The payoff for dedicated 30 minutes per day is well worth the effort. Our skills are constantly eroding, and keeping them sharp is a part of the very definition of “professional.” It is easy to “lose one’s way” in the sense that we get focused on the day-to-day of making money and meeting client demands. Focused reflection and self-study prevent intellectual atrophy. Synthesis: How to Become Influential Find important work, be a good teammate and keep focused on what’s important. To become influential, one must bring these qualities out in others by projecting these traits, through example and encouragement, among your colleagues every day. *Follow Harrison (@5MinuteAnalyst on twitter) and the rest of the CANA Advisors’ Team (@CANAADVISORS on Facebook and twitter) for more insights, blog posts and articles delving into data, logistics and analytics in creative and helpful ways. **MORS is the Military Operations Research Society (MORS). Its focus is to enhance the quality of analysis informing national and homeland security decisions. ***OR/MS Today is a publication of INFORMS.org. For more information on INFORMS or to subscribe to OR/MS Today visit their website at https://www.informs.org/ #MORS #INFORMS #ORMSToday #NPS #excerpt #work #HarrisonSchramm #BrianMorgan #influence #teamwork #collaboration #important
- Does Sports Analytics Help Win Championships?
Over the past four years, a majority of the championship teams from the four major US sports were big users of analytics. So, to the casual observer, the answer must be yes. Now, we would like to prove the case analytically. In 2015, ESPN ranked all MLB, NFL, NBA, and NHL teams and divided the teams into five categories. The article was named “The Great Analytics Rankings.” The first category was “All In.” These teams used analytics to influence team performance at a high level. The next level was “Believers.” These teams were using analytics but not at a high level. The middle level was “One Foot In.” This level represented teams that were testing the analytics waters. The fourth level was the “Skeptics.” This level contained teams with very little analytical capability. The lowest category was the “Nonbelievers.” These teams did not have or did not use the analytical support the team possessed. There are several issues with using the 2015 ESPN analytical rankings. First, over time teams have changed categories. For example, the Philadelphia 76ers were ranked as the number one overall team. Their ranking would have decreased when analytics driven General Manager Sam Hinkie stepped down in April 2016. Then, the 76ers rebounded in January 2017 by adding no less than five highly qualified analytics professionals to their analytics and strategy department. The second issue with the rankings is that ESPN never followed up with another ranking using the same criteria. This second ranking would have helped see changes in the organizational focus on using analytics. Since this analytics ranking is the only one by a major sports media outlet that ranked teams in the four major US sports at one time, we will use it for our analysis. Analysis Setup The analysis took the results of the 2014, 2015, and 2016 seasons and all 122 teams in the ESPN rankings. For analytics rating the following scores were assigned to teams: 5 - All In 4 - Believers 3 - One Foot In 2 - Skeptics 1 - Nonbelievers During the seasons in the analysis, the following scores were assigned to teams: 1 - Qualified for playoffs 0 - Did not qualify for playoffs The following scores were assigned to represent how far in the playoffs teams advanced in the NFL, NBA, and NHL: 1 - Lost in first round 2 - Lost in second round 3 - Lost in third round 4 - Lost championship game 5 - Won the championship game MLB has only three rounds of playoffs (we did not consider the wildcard play-in game a round of playoffs), so the scores were adjusted: 1.3 - Lost in first round 2.6 - Lost in second round 4 - Lost championship game 5 - Won the championship game Analysis Results Here are the 2014-2016 championship teams in each sport and their ESPN analytics score. The average score for all championship teams is four. The only teams below this average were the 2014 San Francisco Giants and the 2015 Denver Broncos. 2014 MLB - San Francisco Giants - 3 NFL - New England Patriots - 4 NBA - San Antonio Spurs - 5 NHL - Los Angeles Kings - 4 2015 MLB - Kansas City Royals - 4 NFL - Denver Broncos - 2 NBA - Golden State Warriors - 4 NHL - Chicago Blackhawks - 5 2016 MLB - Chicago Cubs - 5 NFL - New England Patriots - 4 NBA - Cleveland Cavaliers - 4 NHL - Pittsburgh Penguins - 4 The sports mean and standard deviation for team analytics scores breakout is below. The NFL is the only league with the average score below three. For the teams with an ESPN analytics score of four or five, only thirteen (11% of the total 122 teams) did not make the playoffs any of the three years. This number of playoff teams compares to twenty-eight (23% of the total 122 teams) of the teams with ESPN analytics scores of three or less. The probability of teams with “All In” and “Believers” was 12% higher to make the playoffs than the “One Foot In,” “Skeptics,” and “Nonbelievers.” To compare teams across sports and years with multiple parameters, we used K-Means NCluster analysis. Parameters considered for 2014, 2015, and 2016 seasons were if the team made the playoffs (0-1), how far the team advanced in the playoffs (1-5), and by the ESPN analytics score (1-5). Cluster 1 (red) represents the teams that make the playoffs, advance farther in the playoffs and have higher analytics (3.5). Teams in Cluster 2 (green) have lower analytics (2.9) and much lower playoff performance. Although the differences in the Playoffs and How Far the teams advanced in the playoffs is large for all years, the difference in the analytic rankings is 0.6. K-Means NCluster Table K-Means NCluster Ven-diagram (click image for full size) As with any analysis, the answer is not black and white. Are teams that use analytics performing better and winning more championships? Absolutely! However, the analysis does not prove that analytics is why they are winning championships. The historic performance of the LA Dodgers this year (2017) provides additional evidence. The next question is what team will use analytics to dominate next? *Walt DeGrange is a Principal Operations Research Analyst at CANA Advisors and the INFORMS SpORts Analytics Chairperson currently. To read more on Sports Analytics and article by other members of the CANA Team visit the CANA Blog. #ESPN #WaltDeGrange #INFORMSSpORts #majorleague #sports #analysis #analytics #NFL #NBA #MLB #NHL #hockey #baseball #basketball #football #championshipteams
- What is the best Python IDE?...
So, What is the best Python Integrated Development Environment (IDE)? This question gets asked all the time. The quick answer is... “It depends”. What problem are you trying to solve and where in the CRISP-DM methodology are we operating? Figure 2. CRISP-DM Methodology Some IDEs are better for the Data Understanding and Data Preparation piece while some IDEs are better in the Modeling, Deployment and sharing analysis piece. We actually have three architecture options for Python development – command line, IDE, or Notebook. For tool selection, we need to look at which part of the data science process we are in and how well the tool meets our trade-offs between cost, quality, and time to market. For example, in the data cleansing phase of a project you may just need to use the command line. There are many benefits to this. One great use case for using the command line is maximizing your memory resources with parallel processing for large data sets (see Article by Adam Drake). Python shell scripts work as a great lightweight tool to parallelize existing memory resources. However, if we want to integrate these tools into the data exploration and model-building phase of the projects as well as reuse these tools in other applications – we are going to need an Integrated Development Environment (IDE) for development. IDE’s provide the features for authoring, modifying, compiling, deploying and debugging software. There are a multiple number of IDEs out there and I have experimented with several. I’ve tried Yhat’s Rodeo platform (released after the stackoverflow spreadsheet (Figure 1) was put together), Spyder, PyCharm, Jupyter, and RStudio. I have also done extensive research on stack overflow and various data science blog reviews. My best source however was the Operation Code slack channel. Operation Code is the largest community dedicated to helping military veterans and families launch software development careers. Great content and collaboration for any military veterans transitioning to software development careers. (https://operationcode.org) Here are my thoughts: For Python development and initial code syntax training, you want PyCharm or a similar IDE with Intellisense. PyCharm and Intellisense help new developers with syntax and proper formatting techniques. Intellisense is intelligent code completion and a few IDEs offer this. I was fond of the four Python IDEs that I directly worked with and tested. I thought they were all very easy to use with Yhat’s Rodeo and PyCharm my overall favorites. Yhat has a great data science blog (http://blog.yhat.com) that initially brought me to Rodeo. Ultimately, I had to use PyCharm for a class and stuck with it due to its overall functionality, nice layout, and ease of use. Figure 3: PyCharm Example In Figure 3, our PyCharm example, we see an example of Python code with the yellow highlights indicating Python best practices for syntax. The lines on the right margin indicate severity of the issue by color-coding and where there are conflicts. Yellow indicates a best practice for format tip. If lines to the right were red, we would have a syntax or logic issue causing our code not to run. For data understanding and data preparation, we are going to want something similar to RStudio, Spyder, or Rodeo. The positives with these IDEs are having a variable explorer view so you can see what variables are stored and can double click to view the underlying data and Rodeo automates or at least makes saving the images from graphs very easy. I like RStudio the best due to the ease of use switching between Python, R, and SQL. The ability to move seamlessly between the R and Python in a single environment is particularly useful for cleaning and manipulating large datasets; some tasks are simply better suited to Python, and others to R. One additional benefit to RStudio and Jupyter notebooks is how the code executes in memory. PyCharm, Rodeo, and Spyder have to import packages each time you execute code and some dataframes can take a while to load. With RStudio and Jupyter notebooks it is all in memory so minimal lag time. It is also very easy to share analysis and demonstrate findings. Another great feature of RStudio is the ability to convert notebook and analysis to slides with a simple declaration in the output line: • beamer_presentation - PDF presentations with beamer • ioslides_presentation - HTML presentations with ioslides • slidy_presentation - HTML presentations with slidy • revealjs::revealjs_presentation - HTML presentations with reveal.js Figure 4: RStudio Notebook IDE With ‘reveal js_presentation’ Slide Output My preferred method for new functionality is to develop and test large functions in PyCharm and then move to RStudio notebook for data exploration and building analytics pipelines. You can actually cut and paste Python code directly into R Markdown. All you have to do is tell R Markdown what type of ‘chunk’ to run. For Python: ```{python} … For SQL: ```{r} library(DBI) db <- dbConnect(RSQLite::SQLite(), dbname = "chinook.db") query <- "SELECT * FROM tracks" ``` ```{sql, connection=db, code = query} ``` Note: A future blog post will talk about the convergence in functionality on large datasets between Structured Query Language (SQL) and the R package ‘dplyr’. Figure 5: An example of Python running in an R Markdown document inside the RStudio Notebook IDE For model development and final deployment – here it depends on the size of the dataset and whether or not we will need to use distributed processing with Spark. If we have a large amount of images or any other type of large dataset, we should use Spark’s Databricks platform. Databricks works interactively with Amazon Web Services (AWS) to quickly set up and terminate server clusters for distributed processing. Figure 6. Databricks Notebook Workspace Databricks also automates the install of software packages and libraries to the Amazon cluster greatly decreasing environment setup and configuration time. Figure 7. Databricks Spark Deep Learning Package With the Databricks Community Edition, users will have access to 6GB clusters as well as a cluster manager and the notebook environment to prototype simple applications. The Databricks Community Edition access is not time-limited and users will not incur AWS costs for their cluster usage. The full Databricks platform offers production-grade functionality, such as an unlimited number of clusters that can easily scale up or down, a job launcher, collaboration, advanced security controls, JDBC/ODBC integrations, and expert support. Users can process data at scale, or build Apache Spark applications in a team setting. Additional pricing on top of AWS charges is based on Databricks processing units (DBUs). Figure 8. Databricks Pricing Model (https://databricks.com/product/pricing) Figure 9: Databricks Pricing Example for Production Edition You will need to balance the time saved with Databricks versus the cost of analysts setting up the same environment with other tools but the automated Spark and AWS cluster integration make this a wonderful IDE to work with. Conclusion My top picks... If going to develop a custom algorithm or a custom package in Python – PyCharm If performing data exploration, building analytics pipelines, and sharing results – RStudio If you have a large dataset for Spark distributed processing - Databricks Please comment with your command line/IDE/Notebook best practices and tips. *Jerome Dixon is a valued Senior Operation Research Analyst at CANA Advisors to read more Python articles by him and other members of the CANA Team visit the CANA Blog. #Databricks #RStudio #PyCharm #Spark #DeepLearning #codeexample #Python #IDE #Spyder #stackoverflow #YhatRodeo #CRISPDM
- Make Your Shiny Apps Excel
"Can I view that in Excel?" The capabilities of R programming are expanding. Fast. From publication-quality graphics with ggplot2 to the capability to handle large scale computing with Apache Spark, the analytics community embraces R as a core environment. At CANA Advisors, we use the latest developments in order to deliver the fastest, most adaptable solutions. For clients, results need to be in a form that is easy to process by any member of their team-- with little to no learning curve. As analytics professionals, how can we ensure the best of both worlds? That is, state of the art solutions that produce results in the familiar form clients seek. In this post, I'll go over one such method: using R programming to export the results of a Shiny analysis to Microsoft Excel. For those not familiar with Shiny, it is a package to create interactive, aesthetically pleasing web apps with all the statistical capability of the R programming language. This brief tutorial will utilize the Shiny and XLConnect packages in R. The Method In this example, we'll be working with the iris data set [1], which contains information about the dimensions of different instances of various iris flower species. For the purpose of this tutorial, we'll assume we already have a functioning Shiny app and the data structures we are interested in saving. In this case, the data we'd like to store is reactive in nature. This means, it will change with user inputs. You can recognize calls to reactive expressions in the code below by their distinctive form expression(). To export a worksheet: 1. Lay the groundwork: Create the download button, workbook, and worksheets. 2. Assign the data frames to the worksheets. 3. Save and download. The Result The above process will take us from a shiny app like this: To an excel file like this: The Implementation # Load the shiny and XLConnect packages library(shiny); library(XLConnect) # Create and label the download button that will appear in the shiny app renderUI({ downloadButton("downloadExcel", "Download") }) output$downloadFile <- downloadHandler(filename = "Iris_data.xlsx", content = function(file) # Name the file fname <- paste(file, "xlsx", sep = ".") # Create and assign names to the blank workbook and worksheets wb <- loadWorkbook(fname, create = TRUE) createSheet(wb, name = "Sepal Data") createSheet(wb, name = "Petal Data") # Write the reactive datasets to the appropriate worksheets writeWorksheet(wb, sepal(), sheet = "Sepal Data") writeWorksheet(wb, petal(), sheet = "Petal Data") # Save and prepare for download saveWorkbook(wb) file.rename(fname, file) }) To learn more about any of the features discussed above, use the ?topic feature in R. A more comprehensive overview of shiny is provided by RStudio here. Lucia Darrow is an valued Operation Research Analyst at CANA Advisors to read more R articles by her and other members of the CANA Team visit the CANA Blog. [1] In R, type ?iris to learn more than you would ever want to know about it. #R #Rstudio #shiny #XLConnect #ShinnyApps #codeexample #RStudio #programming #graphics #ggplot2 #LuciaDarrow