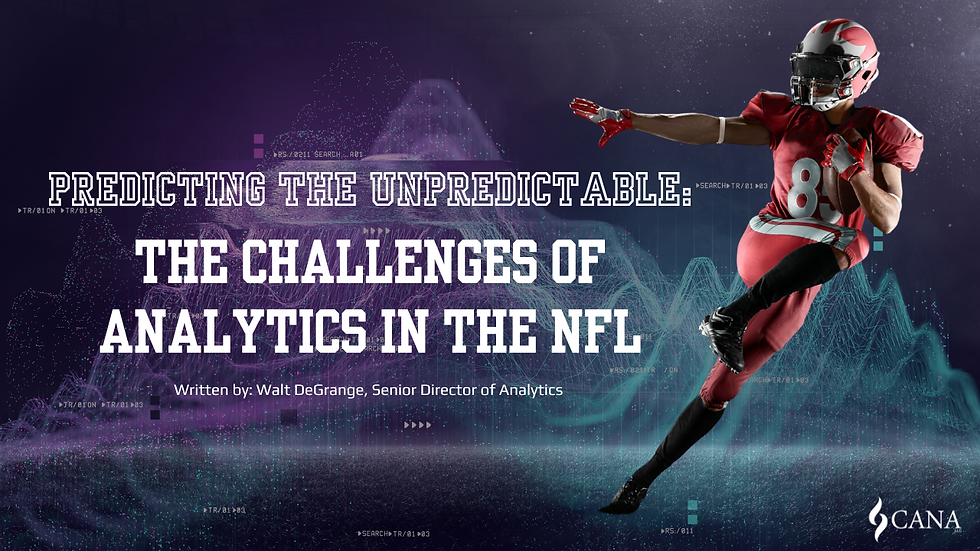
The 2024 NFL season marks 105 years of thrilling gridiron action. With 32 teams playing a grueling 17-game schedule, the league generates a staggering amount of data. Yet, despite this abundance, the NFL presents a unique challenge for data analysts: predicting the unpredictable.
Football, unlike many other sports, is inherently unpredictable. Every play is a new opportunity for momentum to shift, and the outcome can be drastically influenced by a single errant pass or a lucky bounce. The game's unique dynamics, coupled with the limited number of possessions per game, make it challenging to extract meaningful patterns from the data. This unpredictability is further compounded by the constant evolution of strategies and rule changes, which can render previously effective models obsolete.
Compared to other major sports, the NFL plays a relatively small number of games, both in the regular season and the playoffs. This limited dataset poses significant challenges for analysts seeking to develop accurate predictive models. Moreover, the changing nature of the game, with evolving strategies and rule modifications, can render previously effective metrics obsolete.
To address the data limitations, analysts can turn to simulation techniques. By running thousands of simulated games, it's possible to generate synthetic data that can supplement the real-world data and improve the accuracy of predictive models. Simulation can help to create a larger dataset, allowing analysts to explore more complex relationships between variables and develop more robust models.
When working with limited data, there's always the risk of overfitting models to noise or assigning undue importance to metrics that have little impact on the outcome of the game. This is particularly true in football, where the unpredictability of the game can make it difficult to distinguish between meaningful patterns and random fluctuations. It's crucial to approach data analysis with a critical eye and to continually validate the effectiveness of key predictors.
The challenges faced in NFL analytics, such as limited data and the unpredictable nature of the game, are not unique to this sport. Many other analytical problems, particularly in fields like finance, healthcare, and social sciences, also involve dealing with small datasets, complex systems, and the need to predict future events based on limited information. For instance, financial analysts may struggle to predict stock market trends due to the volatile nature of the market and the limited historical data available. Similarly, healthcare researchers may face challenges in developing accurate disease prediction models due to the complexity of human biology and the limited availability of patient data. By understanding the challenges and techniques used in NFL analytics, researchers in other fields can gain valuable insights and apply similar approaches to their own problems.
Key Takeaways for Applying Analytics to Football
Embrace the challenge of limited data: The NFL's unique characteristics present a formidable obstacle for data analysts.
Utilize simulations to augment data: Synthetic data generated through simulations can help overcome the limitations of real-world data.
Continuously validate key metrics: As the game evolves, it's crucial to regularly assess the effectiveness of predictive metrics.
By understanding these challenges and leveraging innovative approaches, data analysts can continue to contribute to the fascinating world of NFL analytics and help teams make informed decisions in the face of uncertainty and, when applied more generally, other challenging business decisions.
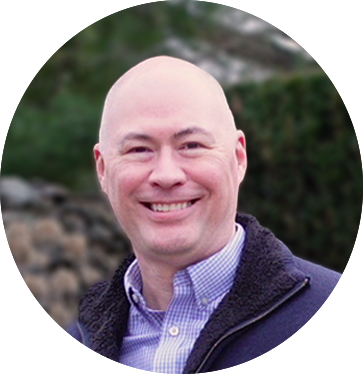
Walt DeGrange
Walt DeGrange is the Senior Director of Analytics here at CANA. You can contact Walt via email at wdegrange@canallc.com or on Linkedin.
Since the agency takes on the responsibility for the guards’ actions as well as their training and compliance with the law california security businesses can mitigate potential legal issues. Additionally, this level of professional oversight can often lead to lower insurance premiums.
Making sure that your business remains relevant and competitive in the long run. With a well crafted strategy in place how do i optimize my arm code for speed you can achieve consistent progress and avoid the pitfalls of short-sighted marketing efforts.
Such as strobing or fading, allows car owners to express their style and creativity. LED strip lights are also popular for next gen ranger light bar accentuating certain features of the car, like the grille or side skirts, making the vehicle stand out.
Instead of solely relying on pharmaceuticals to treat ailments, functional medicine emphasizes the importance of diet, exercise, sleep ozone treatment houston and stress management in achieving optimal health.
It requires a well-rounded strategy that includes SEO, content marketing, social media, email marketing, and more. An agency has the expertise best seo company in texas to develop a cohesive plan that aligns with your business objectives and target audience.